DreamUDF: Generating Unsigned Distance Fields from A Single Image
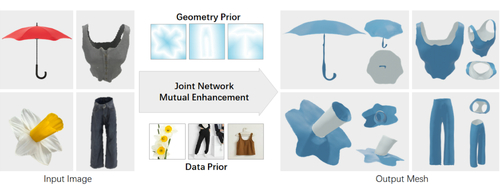
DescriptionRecent advances in diffusion models and neural implicit surfaces have shown promising progress in generating 3D models. However, existing generative frameworks are limited to closed surfaces, failing to cope with a wide range of commonly seen shapes that have open boundaries. In this work, we present DreamUDF, a novel framework for generating high-quality 3D objects with arbitrary topologies from a single image. To address the challenge of generating proper topology given sparse and ambiguous observations, we propose to incorporate both the data priors from a multi-view diffusion model and the geometry priors brought by an unsiged distance field (UDF) reconstructor. In particular, we leverage a joint framework that consists of 1) a generative module that produces a neural radiance field that provides photo-realistic renderings from the arbitrary view; and 2) a reconstructive module that distills the learnable radiance field into surfaces with arbitrary topologies. We further introduce a field coupler that bridges the radiance field and UDF under an novel optimization scheme. This allows the two modules to mutually boost each other during training. Extensive experiments and evaluations demonstrate that DreamUDF achieves high-quality reconstruction and robust 3D generation on both closed and open surfaces with arbitrary topologies, compared to the previous works.
Event Type
Technical Papers
TimeTuesday, 3 December 20249:00am - 12:00pm JST
LocationHall C, C Block, Level 4
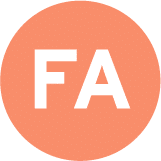
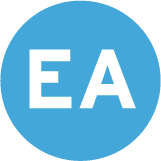
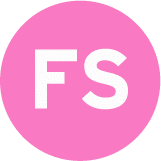
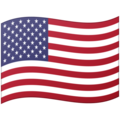