MCNeRF: Monte Carlo Rendering and Denoising for Real-Time NeRFs
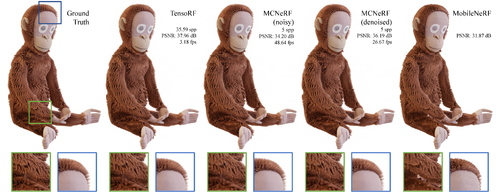
SessionSee Through The Field
DescriptionThe volume rendering step used in Neural Radiance Fields (NeRFs) produces highly photorealistic results, but is inherently slow because it evaluates an MLP at a large number of sample points per ray. Previous work has addressed this by either proposing neural scene representations that are faster to evaluate or by pre-computing (and approximating) scene properties to reduce render times. In this work, we propose \mcnerf, a \emph{general} Monte Carlo-based rendering algorithm that can speed up \emph{any} NeRF representation. We show that the NeRF volume rendering integral can be efficiently computed via Monte Carlo integration using an importance sampling scheme based on ray transmittance distributions. This allows us to, at render time, vary the number of color samples evaluated per ray to trade-off visual quality (noise variance) against performance. These noisy Monte Carlo estimates can be further denoised using an inexpensive image-space denoiser trained per-scene. We demonstrate that \mcnerf can be used to speed up NeRF representations like TensoRF and Instant-NGP by $7\times$ while closely matching their visual quality and without making the scene approximations that real-time NeRF rendering methods usually make.
Event Type
Technical Papers
TimeFriday, 15 December 202310:55am - 11:05am
LocationMeeting Room C4.8, Level 4 (Convention Centre)
