ActRay: Online Active Ray Sampling for Radiance Fields
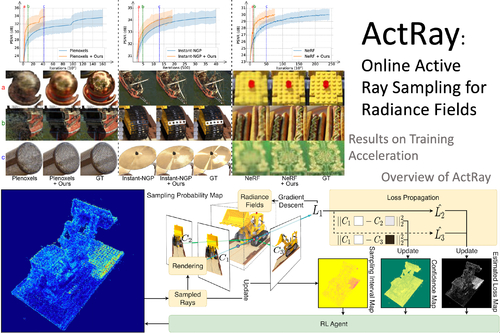
SessionSee Through The Field
DescriptionThanks to the high-quality reconstruction and photorealistic rendering, the Neural Radiance Field (NeRF) has garnered extensive attention and has been continuously improved. Despite its high visual quality, the prohibitive training time limits its practical application. Although significant acceleration has been achieved, it is still far from real-time training, due to the need for tens of thousands of iterations. In this paper, a feasible solution is to reduce the number of required iterations by always training the rays with the highest loss values, instead of the traditional method of training each ray with a uniform probability. To this end, we propose an online active ray sampling strategy, ActRay. Specifically, to avoid the substantial overhead of calculating the actual loss values for all rays in each iteration, a rendering-gradient-based loss propagation algorithm is presented to efficiently estimate the loss values. To further narrow the gap between the estimated loss and the actual loss, an online learning algorithm based on the Upper Confidence Bound (UCB) is proposed to control the sampling probability of the rays, thereby compensating for the bias in loss estimation. We evaluate ActRay on both real-world and synthetic scenes, and the promising results show that it accelerates radiance field training to 6.5x. Besides, we test ActRay under all kinds of representations of radiance fields (implicit, explicit, and hybrid), demonstrating that it is general and effective to different representations. We believe this work will contribute to the practical application of radiance fields, because it has taken a step closer to real-time radiance field training.
Event Type
Technical Papers
TimeFriday, 15 December 202310:45am - 10:55am
LocationMeeting Room C4.8, Level 4 (Convention Centre)
