SIGGesture: Generalized Co-Speech Gesture Synthesis via Semantic Injection with Large-Scale Pre-Training Diffusion Models
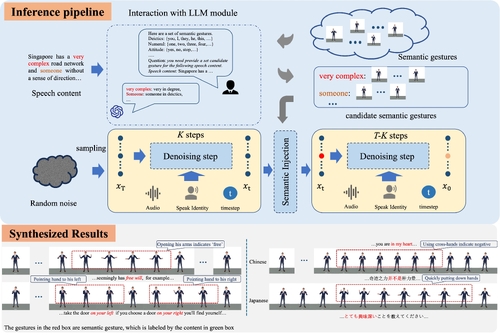
DescriptionThe automated synthesis of high-quality 3D gestures from speech holds significant value for virtual humans and gaming. Previous methods primarily focus on synchronizing gestures with speech rhythm, often neglecting semantic gestures. These semantic gestures are sparse and follow a long-tailed distribution across the gesture sequence, making them challenging to learn in an end-to-end manner. Additionally, generating rhythmically aligned gestures that generalize well to in-the-wild speech remains a significant challenge. To address these issues, we introduce SIGGesture, a novel diffusion-based approach for synthesizing realistic gestures that are both high-quality and semantically pertinent. Specifically, we firstly build a robust diffusion-based foundation model for rhythmical gesture synthesis by pre-training it on a collected large-scale dataset with pseudo labels. Secondly, we leverage the powerful generalization capabilities of Large Language Models (LLMs) to generate appropriate semantic gestures for various speech transcripts. Finally, we propose a semantic injection module to infuse semantic information into the synthesized results during the diffusion reverse process. Extensive experiments demonstrate that SIGGesture significantly outperforms existing baselines, exhibiting excellent generalization and controllability.
Event Type
Technical Papers
TimeTuesday, 3 December 20249:00am - 12:00pm JST
LocationHall C, C Block, Level 4
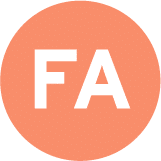
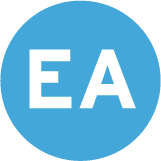
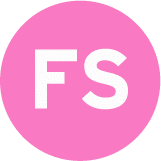
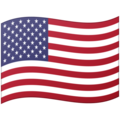