StyleCrafter: Taming Stylized Video Diffusion with Reference-Augmented Adapter Learning
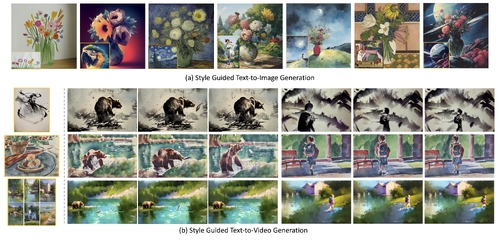
SessionDiffuse and Conquer
DescriptionText-to-video (T2V) models have shown remarkable capabilities in generating diverse videos. However, they struggle to produce user-desired artistic videos due to (i) text's inherent clumsiness in expressing specific styles and (ii) the generally degraded style fidelity. To address these challenges, we introduce StyleCrafter, a generic method that enhances pre-trained T2V models with a style control adapter, allowing video generation in any style by feeding a reference image. Considering the scarcity of artistic video data, we propose to first train a style control adapter using style-rich image datasets, then transfer the learned stylization ability to video generation through a tailor-made finetuning paradigm. To promote content-style disentanglement, we employ carefully designed data augmentation strategies to enhance decoupled learning. Additionally, we propose a scale-adaptive fusion module to balance the influences of text-based content features and image-based style features, which helps generalization across various text and style combinations. StyleCrafter efficiently generates high-quality stylized videos that align with the content of the texts and resemble the style of the reference images. Experiments demonstrate that our approach is more flexible and efficient than existing competitors.
Event Type
Technical Papers
TimeThursday, 5 December 20245:05pm - 5:16pm JST
LocationHall B5 (2), B Block, Level 5
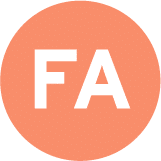
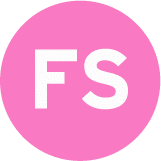
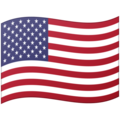