NeuSmoke: Efficient Smoke Reconstruction and View Synthesis with Neural Transportation Fields
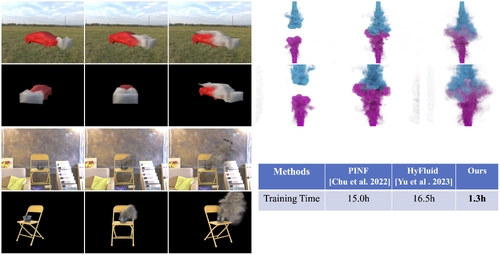
SessionPath Guiding, Scattering
DescriptionNovel view synthesis of smoke scenes presents a challenging problem. Previous neural approaches have suffered from inadequate quality and inefficient training. We introduce NeuSmoke, an efficient framework for dynamic smoke reconstruction using neural transportation fields, enabling high-quality density reconstruction and novel-view synthesis from multi-view videos. Our framework consists of two stages. In the first stage, we design a novel neural fluid field representation, integrating the transport equation with neural transportation fields. This includes adaptive embedding of multiple time stamps to enhance the spatial-temporal consistency of the reconstructed smoke. In the second stage, we combine novel-view color and depth information, employing convolutional neural networks (CNNs) to refine the smoke reconstruction. Our model achieves over 10 times faster than previous physics informed approaches. Extensive experiments demonstrate that our method surpasses existing techniques in novel view synthesis and volume density estimation in real-world and synthetic datasets.
Event Type
Technical Papers
TimeTuesday, 3 December 20245:05pm - 5:16pm JST
LocationHall B5 (2), B Block, Level 5
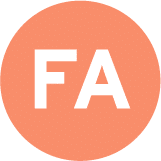
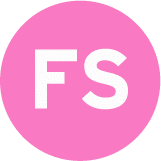
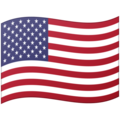