DifFRelight: Diffusion-Based Facial Performance Relighting
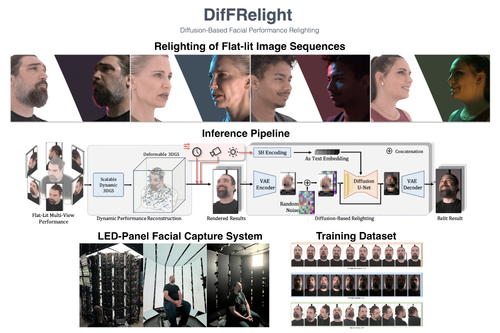
DescriptionWe present a novel framework for free-viewpoint facial performance relighting using diffusion-based image-to-image translation. Leveraging a subject-specific dataset containing diverse facial expressions captured under various lighting conditions, including flat-lit and one-light-at-a-time (OLAT) scenarios, we train a diffusion model for precise lighting control, enabling high-fidelity relit facial images from flat-lit inputs. Our framework includes spatially-aligned conditioning of flat-lit captures and random noise, along with integrated lighting information for global control, utilizing prior knowledge from the pre-trained Stable Diffusion model. This model is then applied to dynamic facial performances captured in a consistent flat-lit environment and reconstructed for novel-view synthesis using a scalable dynamic 3D Gaussian Splatting method to maintain quality and consistency in the relit results. In addition, we introduce unified lighting control by integrating a novel area lighting representation with directional lighting, allowing for joint adjustments in light size and direction. We also enable high dynamic range imaging (HDRI) composition using multiple directional lights to produce dynamic sequences under complex lighting conditions. Our evaluations demonstrate the model's efficiency in achieving precise lighting control and generalizing across various facial expressions while preserving detailed features such as skin texture and hair. The model accurately reproduces complex lighting effects like eye reflections, subsurface scattering, self-shadowing, and translucency, advancing photorealism within our framework.
Event Type
Technical Papers
TimeTuesday, 3 December 20242:45pm - 2:56pm JST
LocationHall B5 (2), B Block, Level 5
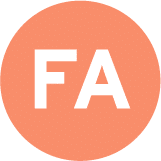
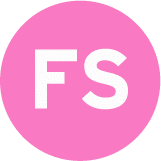
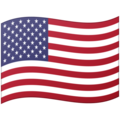