SRIF: Semantic Shape Registration Empowered by Diffusion-based Image Morphing and Flow Estimation
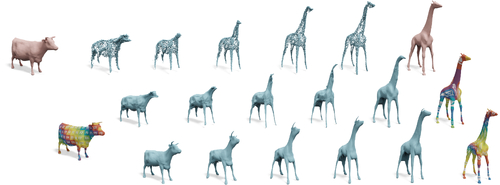
SessionNeural Shapes
DescriptionIn this paper, we propose \textbf{SRIF}, a novel \textbf{S}emantic shape \textbf{R}egistration framework based on diffusion-based \textbf{I}mage morphing and \textbf{F}low Estimation.
More concretely, given a pair of extrinsically aligned shapes, we first render them from multi-views, and then we utilize an image interpolation framework tailored for diffusion models to generate sequences of intermediate images between them. The images are later fed into a dynamic 3D Gaussian splatting framework, with which we reconstruct and post-process for intermediate \emph{point clouds} respecting the image morphing processing. In the end, tailored for the above, we propose a novel registration module to estimate continuous normalizing flow, which deforms source shape consistently towards the target, with intermediate point clouds as weak guidance. Our key insight is to leverage LVMs to \emph{associate} shapes and therefore obtain much richer semantic information on the relationship between shapes than the ad-hoc independent semantic information extraction. As consequence, \textbf{SRIF} achieves high-quality dense correspondences on challenging shape pairs, but also delivers smooth, semantically
meaningful interpolation in between. Empirical evidence justifies the effectiveness and superiority of our method as well as specific design choices. The code will be made public upon acceptance.
More concretely, given a pair of extrinsically aligned shapes, we first render them from multi-views, and then we utilize an image interpolation framework tailored for diffusion models to generate sequences of intermediate images between them. The images are later fed into a dynamic 3D Gaussian splatting framework, with which we reconstruct and post-process for intermediate \emph{point clouds} respecting the image morphing processing. In the end, tailored for the above, we propose a novel registration module to estimate continuous normalizing flow, which deforms source shape consistently towards the target, with intermediate point clouds as weak guidance. Our key insight is to leverage LVMs to \emph{associate} shapes and therefore obtain much richer semantic information on the relationship between shapes than the ad-hoc independent semantic information extraction. As consequence, \textbf{SRIF} achieves high-quality dense correspondences on challenging shape pairs, but also delivers smooth, semantically
meaningful interpolation in between. Empirical evidence justifies the effectiveness and superiority of our method as well as specific design choices. The code will be made public upon acceptance.
Event Type
Technical Papers
TimeThursday, 5 December 20241:58pm - 2:09pm JST
LocationHall B5 (1), B Block, Level 5
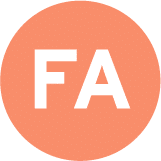
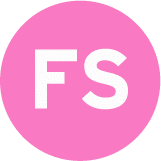
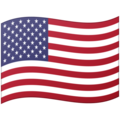