Neural Global Illumination via Superposed Deformable Feature Fields
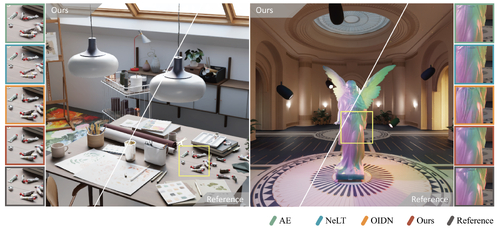
DescriptionInteractive rendering of dynamic scenes with complex global illumination has been a long-standing problem in computer graphics.
Recent advances in neural rendering demonstrate new promising possibilities.
However, while existing methods have achieved impressive results, complex rendering effects (e.g., caustics) remain challenging.
This paper presents a novel neural rendering method that is able to generate high-quality global illumination effects, including but not limited to caustics, soft shadows, and indirect highlights, for dynamic scenes with varying camera, lighting conditions, materials, and object transformations.
Inspired by object-oriented transfer field representations, we employ deformable neural feature fields to implicitly model the impacts of individual objects or light sources on global illumination.
By employing neural feature fields, our method gains the ability to represent high-frequency details, thus supporting complex rendering effects.
We superpose these feature fields in latent space and utilize a lightweight decoder to obtain global illumination estimates, which allows our neural representations to spontaneously adapt to the contribution of individual objects or light sources to global illumination in a data-driven manner, thus further improving the quality.
Our experiments demonstrate the effectiveness of our method on a wide range of scenes with complex light paths, materials, and geometry.
Recent advances in neural rendering demonstrate new promising possibilities.
However, while existing methods have achieved impressive results, complex rendering effects (e.g., caustics) remain challenging.
This paper presents a novel neural rendering method that is able to generate high-quality global illumination effects, including but not limited to caustics, soft shadows, and indirect highlights, for dynamic scenes with varying camera, lighting conditions, materials, and object transformations.
Inspired by object-oriented transfer field representations, we employ deformable neural feature fields to implicitly model the impacts of individual objects or light sources on global illumination.
By employing neural feature fields, our method gains the ability to represent high-frequency details, thus supporting complex rendering effects.
We superpose these feature fields in latent space and utilize a lightweight decoder to obtain global illumination estimates, which allows our neural representations to spontaneously adapt to the contribution of individual objects or light sources to global illumination in a data-driven manner, thus further improving the quality.
Our experiments demonstrate the effectiveness of our method on a wide range of scenes with complex light paths, materials, and geometry.
Event Type
Technical Papers
TimeTuesday, 3 December 20249:00am - 12:00pm JST
LocationHall C, C Block, Level 4
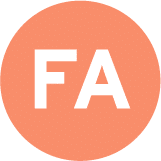
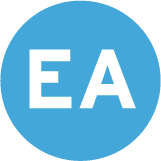
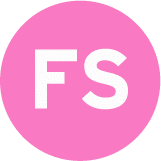
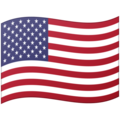